We proposed a new methodology based on NDVI time series autocorrelation values and machine learning algorithms to assess fallowing temporal patterns across rainfed agricultural areas that was tested in mainland Spain. We found that approximately half of rainfed agricultural areas were Regularly Cultivated (RC) and distributed mainly in the north- western Spain whereas lands with crop-fallow rotation patterns every two (CF-2) and three years (CF-3) were distributed across northeast, center and south of Spain. You can see the spatial distribution in the map viewer below. Results were published in Recuero et al., 2019.
Category Archives: Remote sensing data
Spectral Shape Indexes (SSI): calculations using R, an aplication in Andalucía
Palacios et al 2006 first develop angle indexes and explore their utility, since then there have been lots of contributions and aplicactions of this indexes. Some of this indexes are sensitive at tracking moisture and show a temporal profile distinct from spectral indexes, having higher dynamic range and quicker response time to precipitation. As this indexes also show good potential for deriving clear thresholds that discriminate land cover classes.
The aim of this tutorial is the ease the process to calculate angle indexes using the package Angle Indexes develop by GEOQuBiDy. For this example we will used MODIS data and all the porcess will be donde using R.
Load Libraries for the estimation of Angle Indexes
## Libraries
############
library(raster)
rasterOptions(progress = "text", maxmemory = 1e+09)
library(rgdal)
## install and load package to estimate angle indexes for MODIS data
####################################################################
remotes::install_github("klauswiese/AngleIndexes")
library(AngleIndexes)
Load data
#MODIS image from Andalucia
###########################
Andalucia <- stack("https://www.dropbox.com/s/7pom2di45gk9ty6/MODISAndalucia.tif?raw=1")/10000
names(Andalucia) <- c("RED", "NIR", "BLUE", "GREEN", "SWIR1", "SWIR2", "SWIR3")
summary(Andalucia)
#Load political limits of Andalucia
download.file("https://www.dropbox.com/s/lb5dva39b7wc6tu/Andalucia.gpkg?raw=1", destfile = "LimitsAndalucia")
Limits <- readOGR("LimitsAndalucia", "Andalucia")
Mask image and explore data
Andalucia <- mask(Andalucia, Limits) #mask data over the study area
#Plot data
#All bands to be used
plot(Andalucia)
#Composite RGB for the study area
plotRGB(Andalucia, r=1,g=4,b=3, stretch = "hist")
plot(Limits, add=TRUE)
Calculate three of the Angle Indexes: ANIR (Angle in NIR), SANI (Shortwave Angle Normalized Index) and AR (Angle in RED)
#ANIR
ANIR <- ANIRmodis(Andalucia[["RED"]], Andalucia[["NIR"]], Andalucia[["SWIR1"]])
#SANI
SANI <- SASImodis(Andalucia[["NIR"]], Andalucia[["SWIR1"]], Andalucia[["SWIR2"]])
#AR
AR <- ARmodis(Andalucia[["GREEN"]], Andalucia[["RED"]], Andalucia[["NIR"]])
Stack indexes and plot
AngleIndexes <- stack(AR, ANIR, SANI)
names(AngleIndexes) <- c("AR", "ANIR", "SANI")
plot(AngleIndexes)
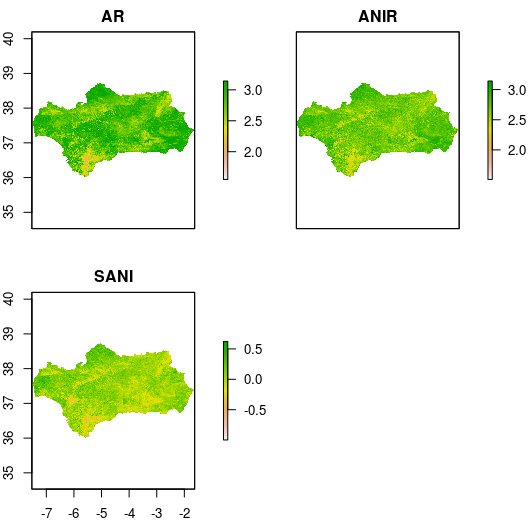
How are vegetation growing seasons around the world?
We identified the most relevant vegetation seasonal patterns based on spectral analysis of NDVI time series. Most of global land surface (93.18%) showed one intra-annual growing season whereas bi- and trimodal patterns occupy lower area (5.58%), mainly in tropical and arid regions along with agricultural areas. The highest values of amplitude and stability were found at Northern Hemisphere high latitudes whereas lowest values were located in tropical and arid regions. High presence of pluri-annual cycles was found in water-limited areas of Australia. See more in this article.
Spectral Shape Indexes (SSI): basis and applications.
In this post we present the basis and possible applications of the spectral shape indices (SSI) which first proposed by members of this research team in 2006 (Palacios-Orueta et al., 2006). These indices are based on the angle formed at any vertex of three contiguous spectral bands in a multispectral broadband spectrum being computed easily by cosine theorem.
Unlike the majority of ratio indices that use two bands , the angles provide information about the relative behavior of the three band set summarizing the shape of the spectrum within their wavelength range. All the angles that we are able to extract from a spectrum of MODIS data are shown in Figure 1-A. Figure 1-B shows the application of cosine theorem to calculate AS1 index derived from NIR (860nm), SWIR1 (1240nm) and SWIR2 (1640 nm) and AS2 derived from SWIR1(1240nm), SWIR2 (1640 nm) and SWIR3 (2130 nm) MODIS spectral bands.
Figure 1: A) Spectral shape and angles located at MODIS bands central wavelength B) Calculation of AS1 and AS2 using the cosine theorem (From Palacios-Orueta et al., 2012)
Depending on the used spectral bands different information regarding to biophysical properties can be extracted. Indices centered in VNIR region provide information about processes related to vegetation pigments such as photosynthesis or greenness. However, indices centered in the SWIR region offer information about the vegetation water content and their application in land surface monitoring is specially interesting when photosynthetic activity is not the dominant process.
Table 1 Spectral Shape Indexes (SSI) proposed. The first column is the name proposed, the second column shows the three spectral bands used to calculate the index, the third column indicates their mathematical value, and the fourth column is the scientific publication in which it has been shown. (From Palacios-Orueta 2012).
Among the results of their application, Palacios-Orueta et al. (2006) showed a good performance of SANI in tracking moisture and discriminating between soil, vegetation and dry vegetation. In addition, they found significant differences between NDVI and SANI in response to precipitation with higher dynamic ranges and faster responses. Khanna et al. (2007) demonstrated that SASI is a good indicator of soil and vegetation moisture whereas ANIR is useful for discriminating dry plant matter from soil. They showed that combining SASI and ANIR indices improves land cover discrimination by highlighting the differences between soil, dry plant matter, and low to high amounts of green vegetation. Huesca et al., 2009 showed the usefulness of AS1 time series to discriminate three vegetation types under different climates. More recently, Palacios-Orueta et al. (2012) assessed AS1 and AS2 behavior over a cotton crop growing period and derived phenological metrics useful to monitor cotton phenological stages. Finally, Tornos et al. (2015) assessed dynamics of SASI and other spectral indices in relation to rice agricultural practices and hydroperiod. They showed that SASI was effectively used to assess variability out of the growing season being able to detect changes in soil water content and to identify the harvest and the end of environmental flooding.
Spectral Shape Indices: application to Doñana and Picos de Europa National Parks
References
- Escribano, P. (2009). Integración de técnicas espectrales para la detección y cuantificación de los tipos de cubiertas en ecosistemas áridos. Bases para un programa de seguimiento de pN Cabo de gata Níjar, Almeria (Doctoral dissertation, Universidad de Almeria).
- Fensholt, R., & Sandholt, I. (2003). Derivation of a shortwave infrared water stress index from MODIS near-and shortwave infrared data in a semiarid environment. Remote Sensing of Environment, 87(1), 111-121.
- Huesca, M., Litago, J., Escribano, P., Palacios-Orueta, A., & Merino-de-Miguel, S. (2009). Utilidad de los índices espectrales AS1 y AS2 para caracterizar tipos de vegetación en zonas bioclimáticas distintas mediante análisis de series de tiempo. In XIII Congreso Nacional de la AET (Calatayud-España).
- Khanna, S., Palacios-Orueta, A., Whiting, M. L., Ustin, S. L., Riaño, D., & Litago, J. (2007). Development of angle indexes for soil moisture estimation, dry matter detection and land-cover discrimination. Remote Sensing of Environment, 109(2), 154-165.
- Palacios-Orueta, A., Khanna, S., Litago, J., Whiting, M. L., & Ustin, S. L. (2006). Assessment of NDVI and NDWI spectral indices using MODIS time series analysis and development of a new spectral index based on MODIS shortwave infrared bands. In Proceedings of the 1st international conference of remote sensing and geoinformation processing (pp. 207-209). Trier, Germany. http://ubt, opus. hbz-nrw, de/volltexte/2006/362/pdf/03-rgldd-session2, pdf.
- Palacios-Orueta, A., Whiting, M. L., Ustin, S. L., Litago, J., Garcia, M., & Khanna, S. (2008). Cotton phenology analysis with the new remote sensing spectral angle indexes AS1 and AS2. In Agricultural and biosystems engineering for a sustainable world. International Conference on Agricultural Engineering, Hersonissos, Crete, Greece, 23-25 June, 2008. European Society of Agricultural Engineers (AgEng).
- Palacios-Orueta, A., Huesca, M., Whiting, M. L., Litago, J., Khanna, S., Garcia, M., & Ustin, S. L. (2012). Derivation of phenological metrics by function fitting to time-series of Spectral Shape Indexes AS1 and AS2: Mapping cotton phenological stages using MODIS time series. Remote sensing of environment, 126, 148-159.
- Tornos, L., Huesca, M., Dominguez, J. A., Moyano, M. C., Cicuendez, V., Recuero, L., & Palacios-Orueta, A. (2015). Assessment of MODIS spectral indices for determining rice paddy agricultural practices and hydroperiod. ISPRS Journal of Photogrammetry and Remote Sensing, 101, 110-124.