Xavier Larriva Novo awarded with the prize to the best Ph.D. thesis work in the fields of defense and security.
Xavier Larriva Novo, a Ph. D researcher at the Universidad Politécnica de Madrid, was awarded the prize for the best doctoral thesis in the fields of defense and security, with the aim of improving individual and collective defense.
The VII CALL FOR AWARDS OF THE “INGENIERO D. ANTONIO REMÓN ZARCO DEL VALLE” awards the best work in specific areas of security and defense. This call takes into consideration several aspects such as the scientific and technological originality of the work, its application to existing challenges in the armed forces, the impact generated, the interest for the Spanish defense and security industry.
The PhD thesis “OPTIMIZATION OF INTRUSION DETECTION SYSTEMS BASED ON MACHINE LEARNING FOR CYBERSECURITY BY BOOSTING FEATURE ENGINEERING AND MODEL SELECTION TECHNIQUES” was developed in the context of intrusion detection systems based on the use of artificial intelligence techniques, especially in the field of machine learning, mainly focused on feature engineering and model optimization. This dissertation contributes to the development of new techniques for intrusion detection. It improves the effectiveness in terms of accuracy by reducing the number of false alarms in the detection of sophisticated attacks generated in real environments.
This Ph.D. thesis work was done mainly in the context of three main projects: PLICA: PLATAFORMA INTEGRADA DE CONCIENCIA CIBERSITUACIONAL; COBRA Cibermaniobras adaptativas y personalizables de simulación hiperrealista de APTs y entrenamiento en ciberdefensa usando gamificación (COBRA), and finally within the European Cybersituational Awareness Platform (ECYSAP) project of the program: European Defense Industrial Development Program.
These projects were carried out in the Redes y Servicio de Telecomunicación e Internet group of the UPM, with Mando Conjunto del Ciber Espacio (MCCE) as the end user.
The main contribution of this PhD thesis in the PLICA project was the machine learning model that allows the application of a characterization to different network scenarios for the detection of cybersecurity anomalies. Based on this model, a distributed pre-processing model for intrusion detection systems was developed for real datasets with real attacks. The result was an intrusion detection system capable of processing large amounts of data with high precision. These solutions made it possible to identify anomalies and attacks in a given scenario with high accuracy.
In addition to this project, this dissertation is part of a second project of the COBRA project program of the Coincidente program, in which the main contribution is in the simulation of Advanced Persistent Threats (APTs), mainly in the development of a formal model that characterizes a parameterizable generic attack, and also in the development of models of known APT infrastructures from existing malware. In this case, based on one of its contributions to intrusion detection, the dissertation contributes in a dynamic way to provide insights for random APT generation.
The third project in which this dissertation is embedded is the program’s ECYSAP project: the main goal of the ECYSAP project is to develop, implement, and integrate theoretical foundations, methodologies, and innovative research prototypes to create a European operational platform that enables rapid real-time cyber situational awareness with defensive response capabilities and decision support for military end-users
This Ph.D. thesis work was tutored by Victor Villagra and Mario Vega Barbas.
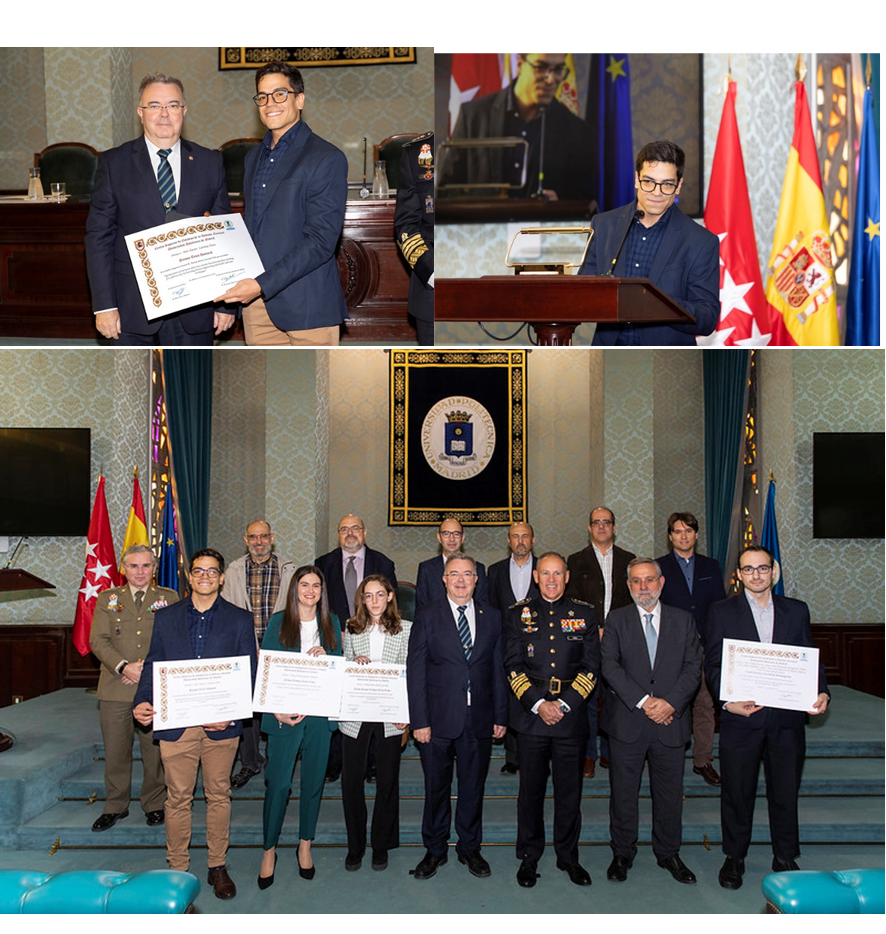